NVIDIA’s rise as a leader in AI hardware is no accident. The company’s GPUs power everything from gaming to data centers, but its dominance in artificial intelligence owes much to collaborations with AI innovators. One such player, DeepSeek, has influenced NVIDIA’s trajectory in subtle but significant ways. This relationship highlights how software advancements and specialized AI research can drive hardware evolution.
The AI Boom and GPU Demand
NVIDIA’s GPUs became the backbone of modern AI because they excel at parallel processing. Training complex neural networks requires massive computational power, and NVIDIA’s chips delivered. But hardware alone doesn’t win markets—software ecosystems and real-world applications do. Companies like DeepSeek, which focus on optimizing AI workflows, pushed NVIDIA to refine its technology for specific use cases.
DeepSeek’s work in areas like natural language processing (NLP) and computer vision relied heavily on NVIDIA’s hardware. By developing algorithms that demanded faster processing and better memory management, DeepSeek indirectly pressured NVIDIA to improve GPU architectures. For example, training large language models (LLMs) requires handling terabytes of data. DeepSeek’s need for efficiency likely influenced NVIDIA’s focus on features like tensor cores and high-bandwidth memory in newer GPUs.
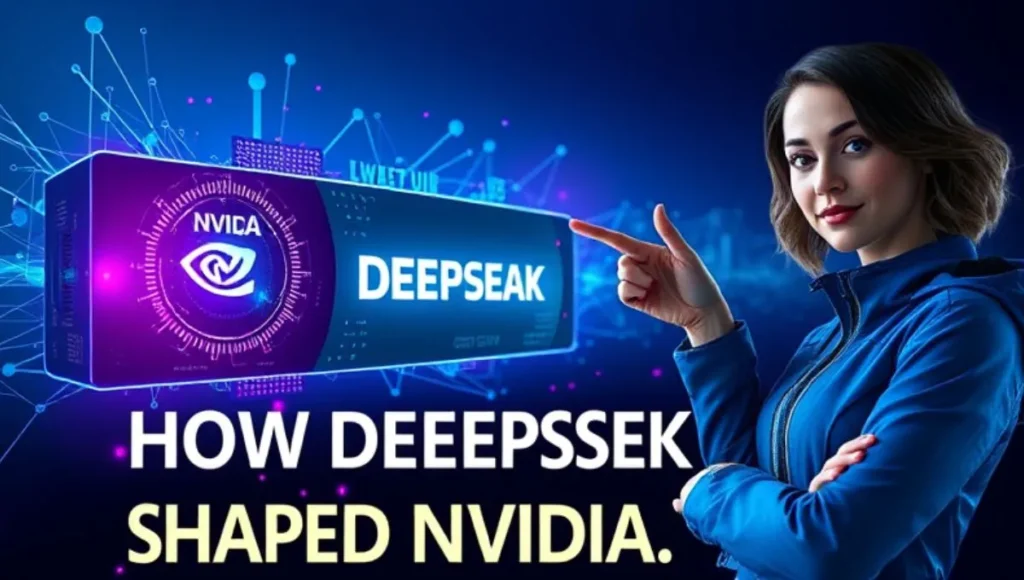
Software-Hardware Synergy
NVIDIA’s CUDA platform, which lets developers harness GPU power for general-purpose computing, became a game-changer. But tools like CUDA thrive when paired with optimized software. DeepSeek’s frameworks and libraries, tailored for AI research, demonstrated how to maximize GPU performance. This feedback loop helped NVIDIA identify bottlenecks and prioritize upgrades.
When DeepSeek released open-source tools for distributed training—a method to speed up AI model development by splitting tasks across multiple GPUs—it showcased the importance of scalable hardware. NVIDIA responded with innovations like NVLink, a high-speed interconnect that lets GPUs share data faster. This advancement benefited the entire AI community, not just DeepSeek.
Related article:
Market Expansion and Competition
DeepSeek’s breakthroughs in niche AI applications also opened new markets for NVIDIA. For instance, DeepSeek’s work on real-time video analysis tools for healthcare and autonomous systems highlighted the need for low-latency, high-performance GPUs. NVIDIA’s Orin and Atlan chips, designed for edge computing and robotics, align with these demands.
Competitors like AMD and Intel have tried to challenge NVIDIA’s AI dominance, but partnerships with firms like DeepSeek give NVIDIA an edge. By collaborating closely with AI developers, NVIDIA gains early insights into emerging needs. DeepSeek’s shift toward energy-efficient AI models, for example, may have influenced NVIDIA’s focus on reducing power consumption in data center GPUs.
Case Study: Optimizing LLMs
Large language models like GPT-4 require weeks of training on thousands of GPUs. DeepSeek’s research into optimizing transformer architectures—a core component of LLMs—revealed opportunities to streamline computations. NVIDIA’s A100 and H100 GPUs incorporated structural changes, such as sparsity acceleration, which cut training times by skipping unnecessary calculations. These tweaks directly addressed pain points highlighted by AI labs like DeepSeek.
The Cost-Performance Balance
AI startups and researchers often face budget constraints. DeepSeek’s efforts to make AI training more cost-effective forced NVIDIA to reconsider pricing strategies. While high-end GPUs like the H100 remain expensive, NVIDIA introduced lower-tier options like the L40S, which offers solid performance for smaller-scale projects. This diversification helps NVIDIA retain customers at all levels, from startups to tech giants.
Ethical AI and Sustainability
DeepSeek’s public stance on ethical AI and reducing carbon footprints also impacted NVIDIA. Training AI models consumes vast amounts of energy, and critics increasingly scrutinize tech companies’ environmental impact. DeepSeek’s push for “green AI” practices likely encouraged NVIDIA to invest in energy-efficient designs, such as GPUs built on TSMC’s 4nm process, which improve performance per watt.
Future Directions
NVIDIA’s roadmap now includes GPUs tailored for generative AI, quantum computing simulations, and climate modeling—areas where DeepSeek is active. The upcoming Blackwell architecture, for example, promises breakthroughs in AI inference speed, a metric critical for deploying models in production. DeepSeek’s feedback during beta testing likely shaped these priorities.
Conclusion
DeepSeek’s influence on NVIDIA isn’t about direct competition; it’s about symbiosis. As AI research pushes boundaries, companies like DeepSeek create demand for better tools. NVIDIA, in turn, evolves its hardware to meet those needs, fueling further innovation. This cycle benefits the broader tech ecosystem, driving progress in AI accessibility, efficiency, and capability.
For NVIDIA, staying ahead means listening to partners who stretch its technology to the limits. DeepSeek represents one of many voices in this chorus, but its impact underscores a larger truth: In the AI race, hardware and software innovators thrive together.